Although machine learning allows organizations to obtain the most optimal solutions to a given problem and enables them to make more precise data-driven decisions, it’s not free from mistakes. Hence, while working on a machine learning project, some pitfalls may occur. In this article, we discuss some ML mistakes and how to avoid them with machine learning experts to help you succeed in your projects.
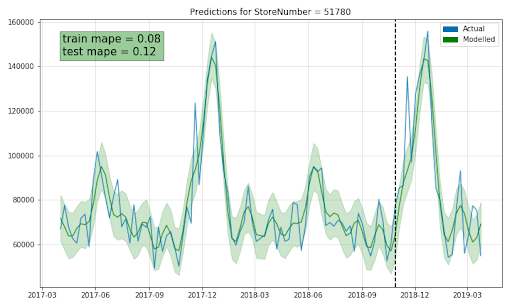
Machine learning can be a bit overwhelming for beginners. The enormous amount of information on this subject can cause some headaches. We hope that this article will make everything at least a bit more straightforward.
Common machine learning mistakes
POOR QUALITY DATA
As machine learning models are tested, trained, and applied to data, data quality is essential – first of all, to get the most accurate results, and is crucial for the algorithms to function properly. Hence, the main issue here is the absence of good data. We can distinguish the following data quality issues:
- Noisy data: This happens when there is a lot of misleading and conflicting information. It results in inaccurate prediction, leading to low-quality results and poor accuracy.
- Dirty data: Contains missing inconsistent and faulty values.
- Sparse data: In this instance, data has very few actual values.
- Incomplete or incorrect data: Incomplete data may lead to faulty programming. In turn, analyzing with less information available may result in less accurate results.
To avoid machine learning mistakes in terms of data, remember to focus on data security, data preparation and integration (format logical for machine learning algorithms), as well as data exploration.
INSUFFICIENT INFRASTRUCTURE FOR MACHINE LEARNING
An adequate infrastructure may prevent the workload and can handle a variety of data that institutions strive to collect and analyze. As a consequence, you should ensure your infrastructure can manage ML. Consider the following areas:
- Flexible storage – develop storage solutions that meet data requirements and allow for future enhancement. When designing storage solutions, usage, data structure, and digital footprint should be considered.
- Powerful computing – data scientists can effectively switch between different data preparation techniques and models by use of scalable, powerful, and secure computing infrastructure. Approaches such as hardware acceleration and distributed computing have proven successful for machine learning.
- Elasticity – the elasticity of infrastructure enables financial expenditures and/or efficient use of limited computational resources.
HAVING NO DATA SCIENTISTS IN THE TEAM
The need for data scientists is growing every month. Why? Nowadays, data plays a crucial role in the business world, and its importance is constantly growing. Many organizations are using it to improve certain aspects of their businesses. If they cannot analyze the data on their own, they have to hire experts.
Most analytics professionals, including data scientists, need a combination of computer science, programming, mathematics, and domain knowledge. The most experienced ones command high fees and expect interesting projects.
THE IMPLEMENTATION OF MACHINE LEARNING TOO SOON OR WITHOUT A STRATEGY
The moment of implementing machine learning is a very important issue. Quite often, organizations decide to incorporate newer modeling methods too soon. In fact, machine learning techniques may not be necessary as in some industries, implementing new models can add a burden.
One of the most daunting tasks is the usage of newer, more complex machine learning strategies with existing procedures. Apart from that, we may distinguish three other issues concerning implementation:
- Lack of data
- Problems with data security
- Long implementation time
In order to solve a given problem, it is necessary to create a strategy for its solution, select the appropriate set of algorithms that will provide the best results.
LACK OF UNDERSTANDING OR SHARING MACHINE LEARNING ALGORITHMS
As machine learning algorithms are complex, they are also difficult to understand. Moreover, most ML algorithms are considered black boxes and thereby present a challenge for many organizations. Among the machine learning mistakes, difficulties in understanding the mathematical aspects of ML algorithms should also be taken into consideration. Mathematics is an important part of machine learning. Ignoring the mathematical treatment of algorithms may lead to the adoption of a limited interpretation of the algorithm and the use of ineffective optimization algorithms.
We’ve discussed five common machine learning mistakes. From today, you know how to avoid them in your own business when working on a machine learning project.
To use ML effectively in your business, you should:
- Understand how the technology works as a part of a broader analytics environment
- Familiarize yourself with a proven application of ML
- Anticipate the challenges you may face
- Learn from industry leaders
ML is an important process and a rapidly growing industry that can boast advanced technological achievements. So it’s no surprise that many industries are taking advantage of machine learning to help with big data analytics and other projects. If you want to know more, take a look at Addepto’s machine learning consulting services.